Artificial Intelligence (AI) and language models have become a large part of the zeitgeist in the last year. Whether it be in the education, pharmaceutical, or supply chain sector, there is a use for AI. For those who need a refresher, AI is the theory and development of complex computer systems that are able to perform tasks that require human intelligence, like decision-making, speech recognition, and visual perception. In the supply chain space, AI is used to perform a variety of tasks already, such as:
- Intelligent demand forecasting and inventory optimization
- Predictive analytics for maintenance and equipment optimization
- Enhanced order fulfillment and logistics
- Robotics and automation in warehousing
- Real-time Warehouse Visibility and Asset Tracking
- Predictive Analytics for Supply Chain Optimization
- Continuous Improvement and Process Optimization
But as is the case with most technologies and improvements, it’s not all milk and honey, there are important considerations to be aware of, a big one being ethics.
Ethical considerations of AI in warehousing
AI processes copious amounts of data to make decisions, which means the data it receives informs everything it does. Therefore, if the input data is biased, then its decisions will be biased too. This is an issue that we are already facing, with some AIs having stronger racial and sexist biases than some humans show unconsciously.
But these biases go beyond social issues. A bias in the warehousing sector could be related to vendor selection. For example, the AI may opt to select suppliers or locations based on historical data — if the data only shows three locations historically used, the AI won’t consider new locations that may be more valuable down the line. This could lead the operation to have inefficient resource allocation and impact future endeavors.
Privacy and data security are also a concern. For example, a company using AI to track consumer behavior would quickly identify patterns of stockouts for specific products. They could then use that data to create artificial scarcity to promote comparable products with higher profit margins. Clearly, this unethical behavior would chip away at the trust between businesses and consumers.
These are important to consider now, before AI is fully implemented. As supply chain leaders and adopters of burgeoning technology, the onus is on us to implement rigorous data protection measures to foster transparency, fairness, and responsible use of AI. This is where human oversight in collaboration with AI comes into play. Supply chain professionals should be actively involved in defining AI objectives, setting boundaries, and providing updated data so that the mechanism is working with the cleanest set of data possible. This leads to another, just as necessary consideration, data quality.
The importance of data quality
The data input into the AI has a direct impact on the performance, accuracy, and reliability of the AI model. The better the data, the better the predictions, which will produce better outcomes and thus more confidence in the AI’s ability to be of value. It’s a fairly simple concept, if you put bad data in, you’ll be getting bad data out.
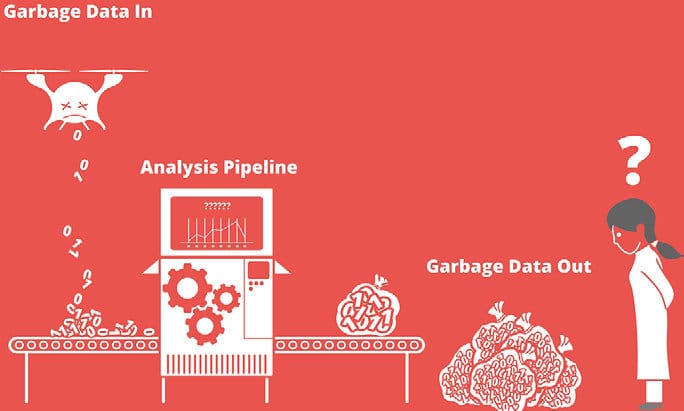
This is especially important for AI use in a warehouse setting using AI for predicting demand, process optimization, and training. To avoid using potentially biased or compromised data, you’ll want to engage in data cleaning, preprocessing, and augmentation of data in addition to implementing consistent and robust evaluation metrics to assess the AI performance regularly. When selecting the data to input into the AI, the main components of quality to look for are:
- Accuracy
- Consistency
- Completeness
- Timeliness
- Relevance
Collecting data can be difficult to do, especially if it’s coming from various sources. There are certain measures one can take to ensure the best data quality in AI, like implementing data governance policies, using data quality tools to automate cleansing and validation, continuously monitoring data quality metrics, and developing a data quality team dedicated to ensuring quality. Remember, the more data the AI has, the better, but if it’s bad data, then it won’t be of any use. When it comes to AI, quality and quantity are both critical.
The amount of data the supply chain has access to is staggering. And while the supply chain uses automation effectively to improve productivity, it is not yet fully optimized for flow, for that, suppliers would need to see themselves as partners, not competitors.
Data sharing challenges
In an ideal supply chain ecosystem, all players — manufacturers, carriers, shippers, and drivers — would work together. Clean and robust data gathered from all segments of the supply chain and compiled into a single system would grant the greatest visibility, fostering collaboration and unity between all participants. However, most companies will balk at sharing any data, especially not with an entity they may consider a competitor.
While that magnitude of data sharing may not be possible today, it’s not unlikely. With the proliferation of so many options out there, it is likely that soon there will be a way for organizations to safely share their data without showing their hands. For now, as supply chain leaders, it’s in our best interest to use AI responsibly and with the contrast reminder that it is a tool, not a solution.
In Summary
The integration of AI into the supply chain holds immense promise for revolutionizing efficiency and productivity. Striking a balance between quantity and quality of data is crucial, and working through the myriad of ethical considerations won’t be easy, but is essential.
Clean data has been the foundation of every project Cornerstone Edge has worked on. It’s what our 3D methodology is built upon. Are you looking to make your supply chain more efficient? We‘ll take a data-centric approach to your needs and custom-build a solution that will propel you forward. Give us a call, and let’s see how we can improve your tomorrow.